How do we leverage the human advantage to make human thinking visible in the age of cognitive technologies?
The End of High School English? Or, Photography's Not Dead!
The question of assessment practices in the context of artificial intelligence is on a lot of educators’ minds. Humanities and English teachers, for instance, have expressed their wonders and worries about generative Large Language Models, like ChatGPT, and the media frenzy that followed OpenAI's release of GPT-3 hasn't helped as well, with some pondering whether this signals the end of the high school English class or the sudden death of the classic college essay.
Questions worth exploring, for sure, but perhaps in less dramatic terms.
What I'd like to explore here feels less dramatic, I hope, but urgent nonetheless:
How do we distinguish human cognition from the work of machines? How do we make human thinking visible and therefore something we can assess with confidence?
A secondary question, connected to this, that worries many teachers is why would students choose to outsource their work to automated machines?
First off, the reality is that teachers and students have been playing “the imitation game” well before AI became publicly accessible like it is now. Am I assessing this student’s cognitive work, or did they read this online? Are students simply aping back what they heard the teacher say? Is that ok?
Ultimately, we have to define more precisely what kind of cognition we want to assess. Put another way, what's the competency we wish to measure?
If it’s recall, perhaps imitating the teacher is perfectly suited to the assignment. I discussed this in an earlier post, reminding readers that writing is a technology, not a competency. And yes, writing has, to some extent, been cognified, much like the digital camera from years previous. As Kevin Kelly writes, “...photography [well before writing] has been cognified. Contemporary phone cameras eliminated the layers of heavy glass by adding algorithms, computation, and intelligence to do the work that physical lenses once did. They use the intangible smartness to substitute for a physical shutter” (2016, 34). Despite this, there are still photography classes to be taught in the age of AI, meaning human creativity and ingenuity are still valuable and necessary parts of the artistic process. Technology did not signal "the death of photography" in schools.
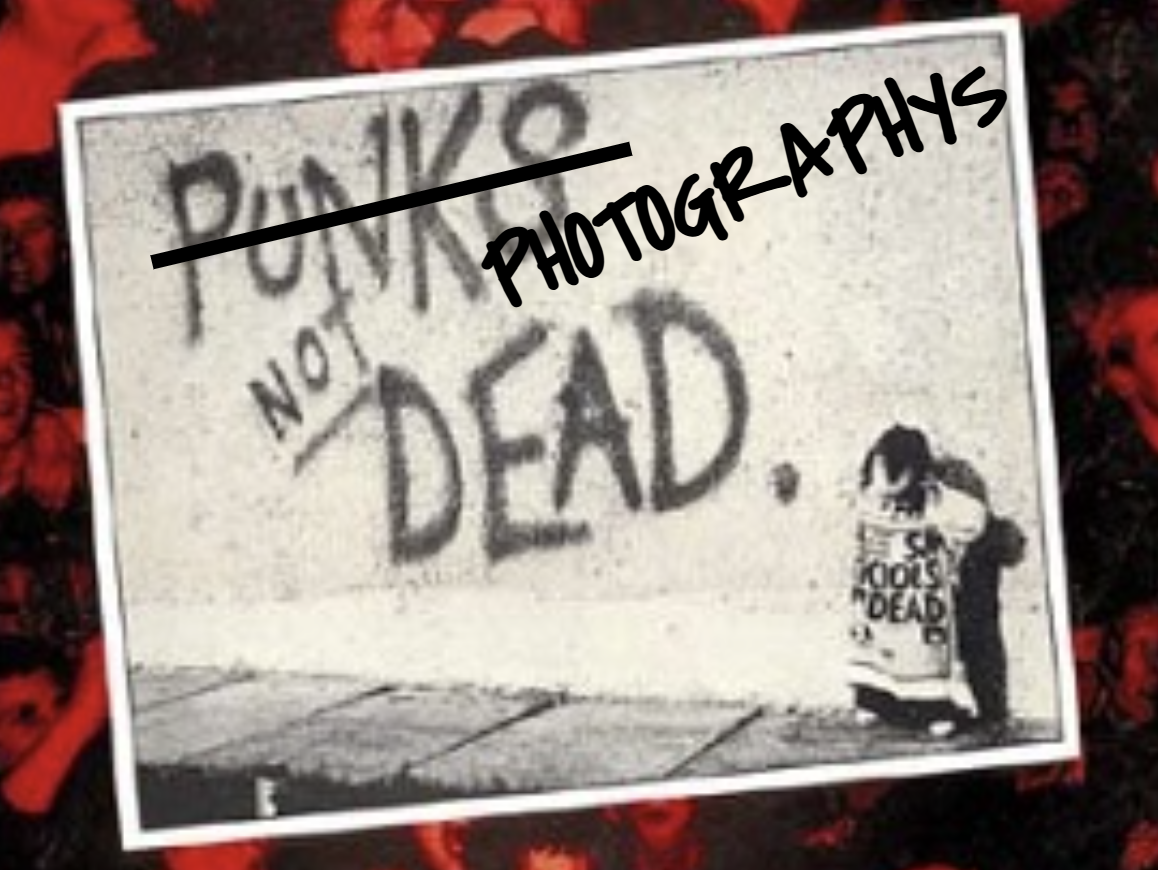
The question, then, is not whether writing is dead (it's not!) but for what reason are we doing it and at what level of complexity (in terms of the greater context). After all, complex communication will be an important human competency in the age of intelligent machines.
Vigorous Complexity over Rigorous Difficulty
A tool that can help clarify matters related to measuring human cognition is Norman Webb’s 4-Level Depth of Knowledge framework, level one being recall and reproduction; level two, skills and application (comparing, summarizing, estimating, etc.); and levels three and four being reserved for more complex strategic and extended thinking, respectively.
In our current moment of technological progress, AI programs have superhuman ability when it comes to level one, and they prove to be pretty impressive in most cases when replicating the skills represented by Webb's level two. For AI programmers, “the difficult part of knowledge is not stating a fact, but representing that fact is a useful way” (Hawkins 2022, 120).
It’s the difference between knowing “what” and knowing “what if,” which scales perfectly with Webb’s four level framework. “What if” is a cognitive maneuver that demands us to extend our thinking in ways that robots cannot do very well because they operate by statistics, not by well-informed, imaginative speculation grounded in a model of a world that's informed by lived experience. It may seem like generative AI models (like ChatGPT) have made it more difficult to see and determine whose cognition we are assessing, but from another perspective, the dust has settled all the more definitively in the era of AI because one thing is clear: certain DOK levels are more suited to authentic, human intelligence versus the ones that are easily performed by artificially intelligent machines. It is not about what you know; it is about what you do with what you know, and humans can do things that machines cannot. It's also not so much about rigor and difficulty, as it is about the vigor demanded of humans when faced with the complexity of a challenge or task (Webb 1, 2021).
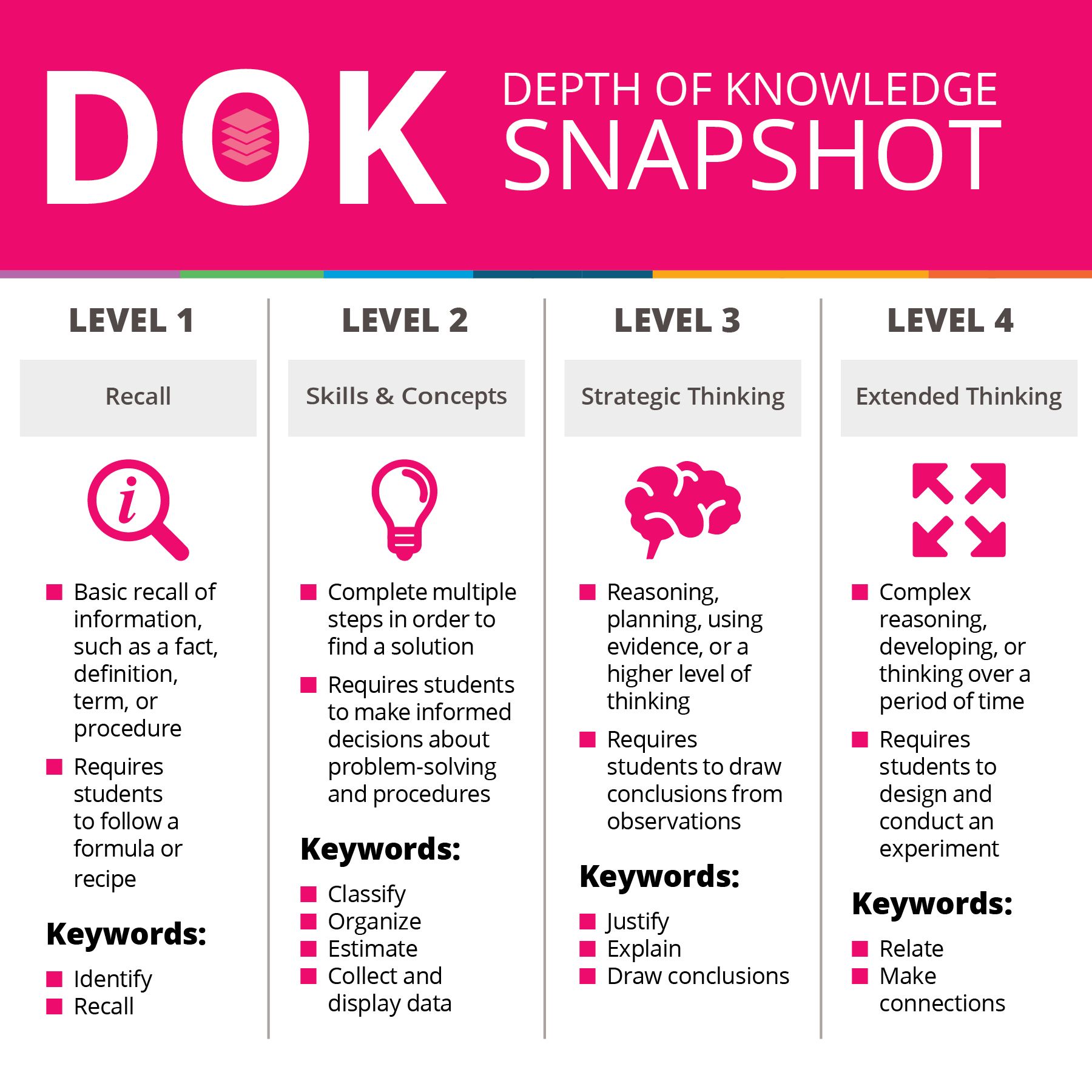
The Human Advantage is about Complexity
In my last post, I referenced the Human Advantage - namely the fact that humans have common sense, background knowledge, and lived experience. We're embodied and able to recognize visual situations, and we reflect metacognitively while also knowing how to interact with others in ambiguous situations. As humans, we know there is a world, and we defer to it while also constantly adjusting our mental models to map its infinite complexity.
Knowing we have this advantage, it is necessary to keep in mind that machines demonstrate "artificial" intelligence, abeit superhuman at times and able perform some of the most difficult tasks (like beating Garry Kasparov at chess), but intelligence that pertains to specific, narrow tasks, whereas what makes human intelligence "authentic" is that we can learn anything. In other words, we know how to navigate complexity.
And there's a lot that AI is missing. In How We Learn: Why Brains Learn Better Than Any Machines... For Now (2020), Neuroscientist Stanislas Dehaene lists some of these shortcomings (for now), some of which I provide here:
- Learning abstract concepts: “[machines] actually tend to learn superficial statistical regularities in data, rather than high-level abstract concepts… human learning is not just the setting of a pattern-recognition filter, but the forming of an abstract model of the world” (28-29).
- Data-efficient learning: “machines are data-hungry, but humans are data efficient. Learning, in our species, makes the most from the least amount of data” (30).
- One-trial learning, or the ability to “integrate new information within an existing network of knowledge” (31).
- Systematicity and the language of thought, or “the ability to discover the general laws that lie behind specific cases” (31).
- Composition: "[In current neural networks,] the knowledge that they have learned remains confined in hidden, inaccessible connections, thus making it very difficult to reuse in other, more complex tasks. The ability to compose previously learned skills, that is, to recombine them in order to solve new problems, is beyond these models… In the human brain, on the other hand, learning almost always means rendering knowledge explicit, so that it can be reused, recombined, and explained to others” (33).
- Inferring the Grammar of a Domain: “Finding the appropriate law or logical rule that accounts for all available data is the ultimate means to massively accelerate learning–and the human brain is exceedingly good at this game” (35).
What all this means is that human thinking can be made explicitly visible if we invite students to do work complex enough to be worthy of our unique, organic cognitive capacities. Based on this research, I recommend the following indicators as a way to verify and evaluate an assessment or performance task's level of complexity:
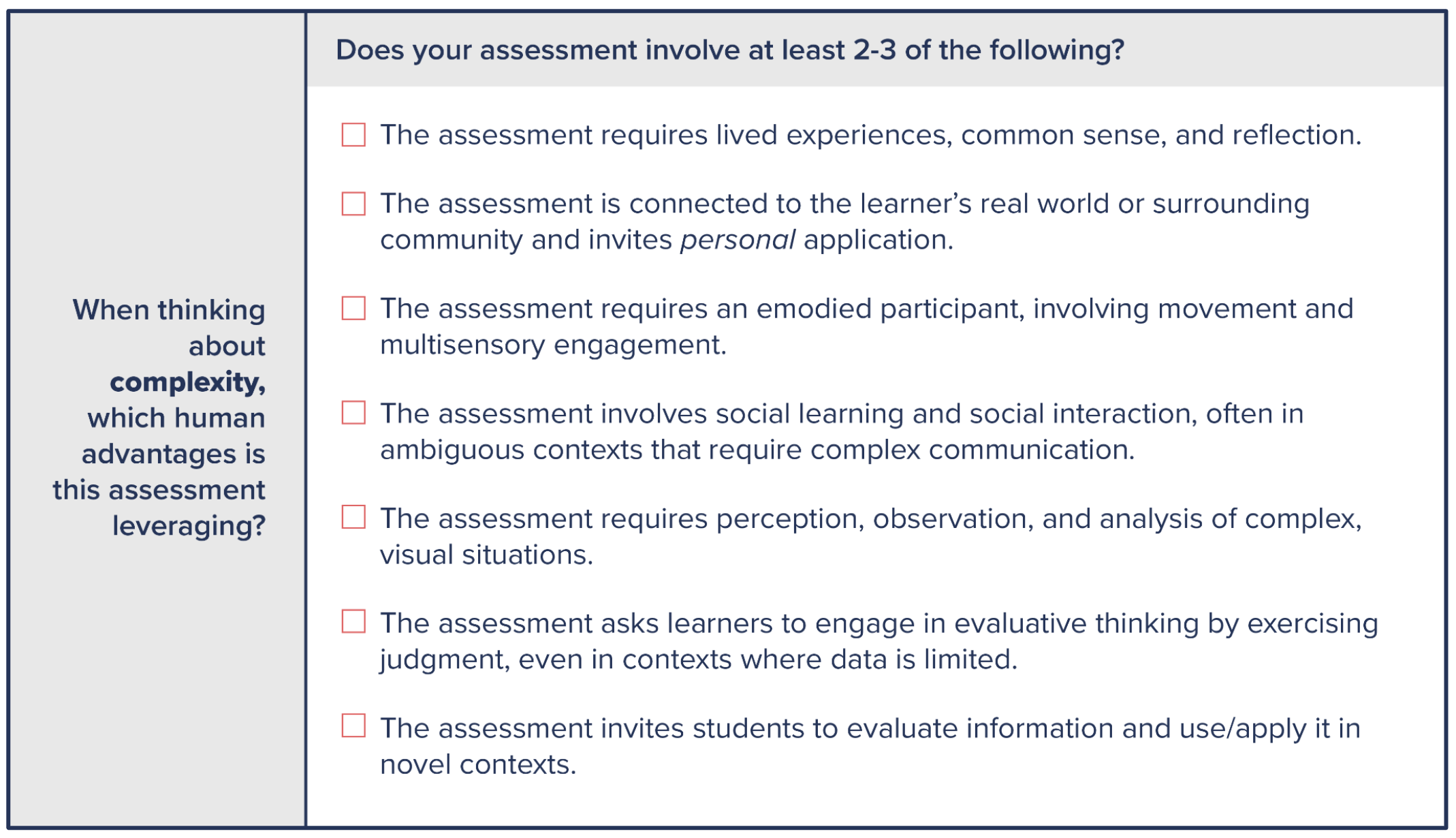
It's important to note that I am not suggesting that all of these boxes must be checked, but if an assessment incorporates at least 2 or 3 of these, then perhaps we're dealing with a complex task that requires the human touch.
To Automate or not to Automate, That is the Question:
When asking the question, why would a student choose to outsource their work to machines?, the complexity of the assignment is only one aspect of the matter.
Consider the following four necessary components for nurturing healthy engagement in learners:
- A safe sense of belonging
- A feeling of competence
- A clear sense of meaning
- A feeling of purpose
One reason a student may outsource their work is because they don't believe they can do it (competence) or they sense that others don't believe in them (belonging). I've written about the connection between competence and wellness in other contexts. Another reason, however, is that the task or assignment might lack meaning or purpose from the perspective of the learner: they don't see "the why" or the reason for doing the work and therefore have no ownership of the endeavor. That's why I recommend considering these elements when reviewing the design of an assessment, assignment, and/or project:
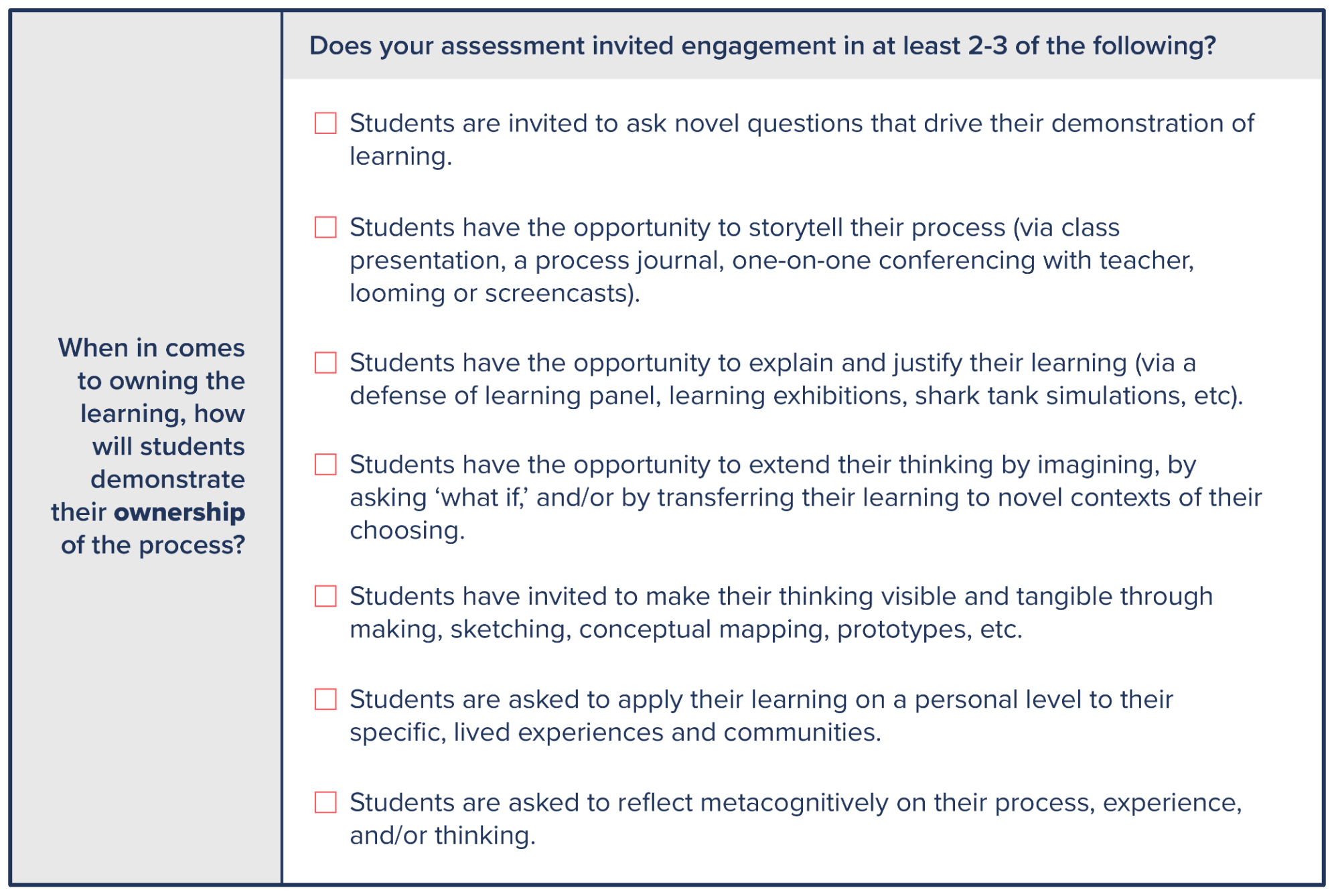
Notice there's overlap from the former list, and like the previous suggestions, the idea is not to check every box here, but to consider whether the learning opportunity involves 2 or 3 of these elements. If so, most likely there's a clear invitation for student ownership, for meaningful and purposeful pursuit of excellence, which will look different for every child.
The point here is not to fool-proof assessments such that artificial intelligence has no part to play. The point here is to remind us of what good assessments and good performance tasks have always looked like. In fact, in no way would I want to suggest that technology has no part to play, but how might we be intentional about its role? When working with students on action plans for completing a task or project, consider returning to the SAMR model in the following way:
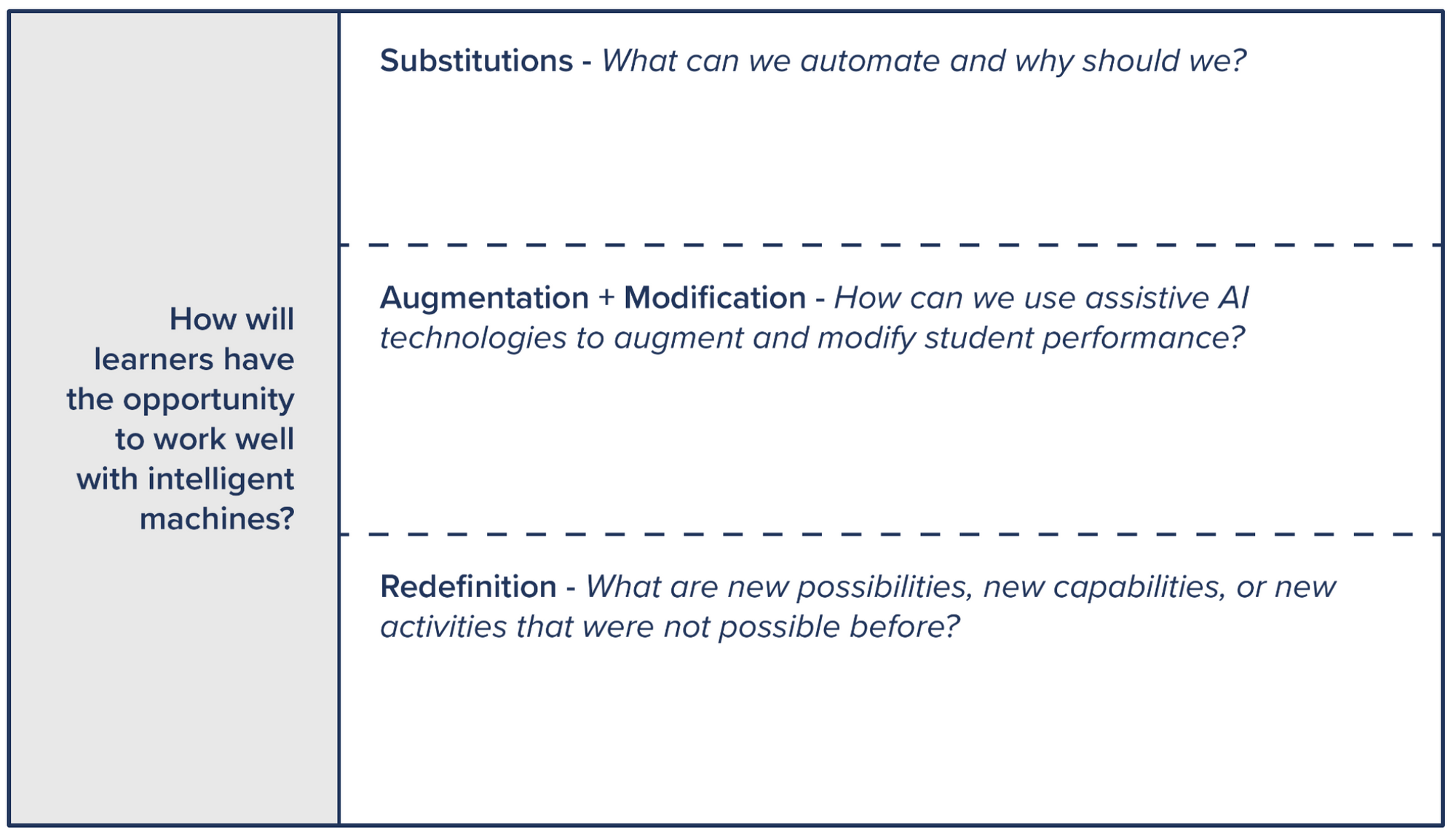
When asking this question, make sure to personalize it. To know how AI can augment and modify an individual's performance, we must know the individual. An "achiever," who seeks to maximize results while optimizing efficiency, may work well with machines in very different ways than an "explorer" who seeks to search, experiment, and discover at a very different pace.
Also, remember to ask the question, what competency do we seek to nurture and measure? (I wrote about this previously in the context of writing) The answer to this question grants us guidance on knowing when to seek the assistance of AI and when not to, which requires the teacher's judgment and professional discretion.
And lastly, let kids formulate the driving questions whenever at all possible. Intelligent machines can provide brilliant answers to challenging questions, answers that sometimes surprise us in thrilling ways. What AI cannot do well, however, is ask truly novel questions that inspire us to explore the unknown. There is probably an AI system that could answer any question about the known cosmos, for instance, but no intelligent system would ever have posed the following imaginative question, a question I once heard Christian Long pose when keynoting a conference years ago: What if we built the Hubble Telescope and simply pointed it into darkness? What might happen? Humans have the curiosity and audacity to ask new, and often rediculous, questions that inspire us to create and construct knowledge and meaning; AI merely reflects back to us both the ingenuity of our inquiries as well as the biases through which we've asked them.
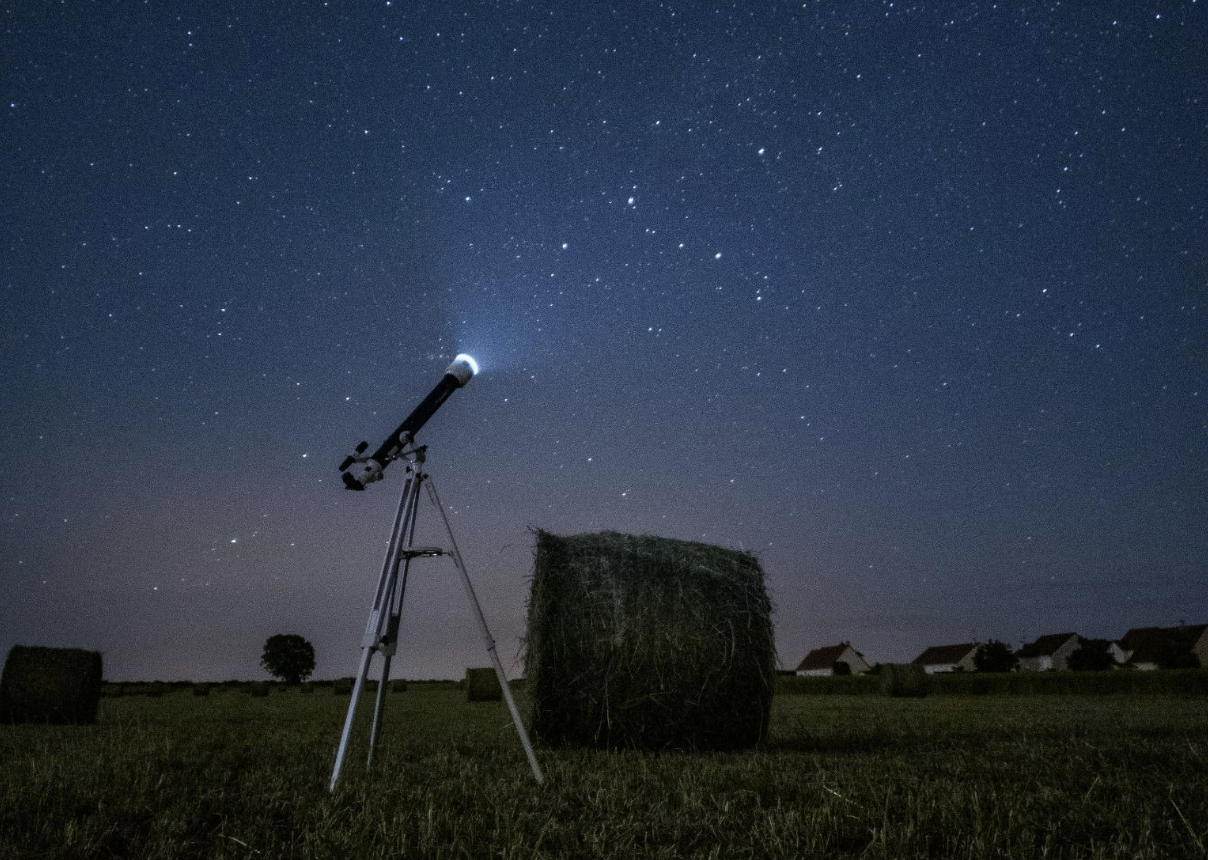
Resources:
- Developing Assessment Complexity: A Tool (created by Jared Colley)
- A Guide to Performance-Based Assessment Development (adapted by Jared Colley from Karin Hess, Rose Colby, and Daniel Joseph’s Deeper Competency-Based Learning: Making Equitable, Student-Centered, Sustainable Shifts, Corwin, 2020)
- Artificial Intelligence + Academic Integrity - a Resource for Teachers (created by Jared Colley)
Sources:
- Daniel Herman. “The End of High School English.” The Atlantic. The Atlantic Monthly Group, 9 December 2022. Accessed 13 February 2023. https://www.theatlantic.com/technology/archive/2022/12/openai-chatgpt-writing-high-school-english-essay/672412/.
- Stephen Marche. “The College Essay Is Dead.” The Atlantic. The Atlantic Monthly Group, 6 December 2022. Accessed 13 February 2023. https://www.theatlantic.com/technology/archive/2022/12/chatgpt-ai-writing-college-student-essays/672371/.
- Gerald Aungst. “Using Webb’s Depth of Knowledge to Increase Rigor.” Edutopia.org. George Lucas Educational Foundation, 4 September 2014. Accessed 2 March 2023. https://www.edutopia.org/blog/webbs-depth-knowledge-increase-rigor-gerald-aungst.
- Kevin Kelly. The Inevitable: Understanding the Twelve Technological Forces That Will Shape Our Future. Viking Press, 2016.
- Jeff Hawkins. A Thousand Brains: A New Theory of Intelligence. Basic Books, 2022.
- Norman Webb. DOK Primer. WebbAlign, 2021. Accessed 30 October 2023. https://www.webbalign.org/dok-primer.
- Stanislas Dehaene. How We Learn: Why Brains Learn Better Than Any Machine... For Now. Penguin Books, 2020.
- Ruben R. Puentedura. “Transformation, Technology, and Education.” Strengthening Your District Through Technology Workshop, 18 August 2006. Accessed 14 March 2023. http://hippasus.com/resources/tte/.
- Parts of this post were adapted from the following: Jared Colley. A People-Centered Organization Living in an AI World, Transformation R&D Report, Vol. 1. Ed. Dr. Brett Jacobsen, MV Ventures, Summer 2023. [Purchase and download a copy here]
Comments